Tech Applications: Fin. Fraud Prevention & Risk Control Analysis
At present, digital technology, while promoting the high-quality development of China's real economy, has also become a catalyst for the growing black and gray industries, leading to increasingly rampant fraud crimes, with telecom network fraud being a representative example.
As the main body responsible for anti-fraud, financial institutions have been implementing various measures to combat and control fraud, establishing and improving risk monitoring and interception mechanisms, and evolving their anti-fraud systems from single system modules to enterprise-level risk control platforms.
This has significantly enhanced their risk monitoring capabilities and gradually enriched and improved the industry ecosystem.
The Party Central Committee places great importance on combating and governing fraud crimes.
GeneralHere is the translation of the provided text into English: 1.
The formation of the dark and grey industry chain has led to an escalating difficulty in defense and attack.
Currently, fraud is characterized by concealed methods, diversified technical tactics, scenario-based behaviors, and high-frequency incidents.
Advertisement
In terms of industry ecology, the dark and grey industry collaborates closely with upstream and downstream partners, making the fraud links more covert and difficult to identify and combat.
For instance, the dark and grey industry spreads various false advertisements for debt evasion and credit repair through the internet, aiding borrowers in using various irregular means to escape debts.
This results in organized and premeditated debt evasion behaviors.
Compared to source governance, merely targeting the borrower's actions cannot achieve a comprehensive effect.
In terms of hardware, attackers in the dark and grey industry use devices such as cat pools, SMS sniffers, and VOIP/GOIP internet telephony equipment to tamper with proxy IPs, disguise IP addresses, change machine brushing, and alter mobile device attributes to counter cluster analysis.
They set up peculiar characters, homophones, and professional jargon to combat voice and semantic recognition.
They develop "universal faces" to counter biometric recognition, causing great trouble for financial institutions.
On the technical front, from traditional hacker attacks, phishing websites, and Trojan viruses to emerging technologies like deep fakes and biometric information theft, the dark and grey industry's technological applications are continuously updated and upgraded, making it difficult for financial institutions to identify and guard against them.
Moreover, as financial institutions increase their defense efforts, the methods used by attackers, such as fraud scripts, bank card sales, and funding channels, have also undergone corresponding adjustments.
The financial products affected are becoming broader, greatly increasing the difficulty for financial institutions to identify and control.
2.
Data barriers are difficult to break down, making it impossible to gain a comprehensive view of fraud.
First, data within financial institutions lack fluidity and sharing.
Inconsistencies in internal data standards or closed data interfaces; differences in the use, structure, value, and quality levels of data from different businesses and periods all increase the difficulty of data collection and integration.
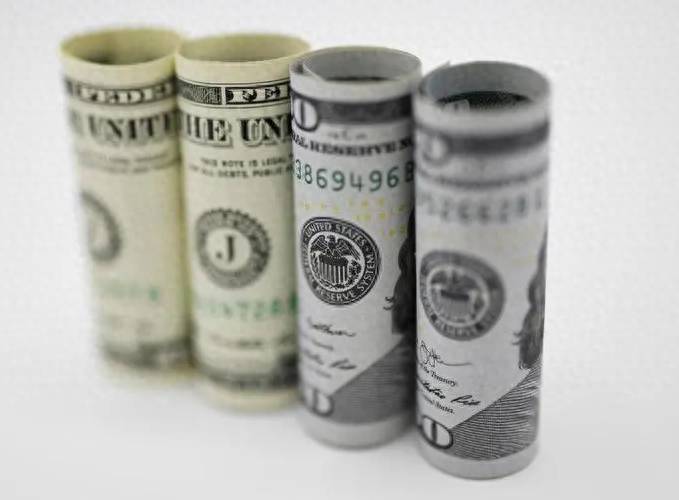
This ultimately results in fraud-related transactions in different business systems being difficult to detect due to data dispersion.
For example, a fraudster who took out a loan for Product A a year ago and defaulted can still successfully obtain Product B from the same financial institution.
Second, there is a lack of data sharing and circulation between institutions and even industries.
A complete fraud process involves multiple entities such as operators, financial institutions, and internet platforms.
It is difficult to detect cross-institution or cross-domain fraud with only partial information held by individuals, putting financial institutions on the defensive in the fight against the dark and grey industry and facing the problem of asymmetric information with criminals.
3.
There are still issues with model applications, and manual verification has not yet been replaced.
First, model performance is easily affected by data quality.
Currently, financial institutions' fraud feature recognition technology mainly relies on data-driven models, which require sufficient data and computing power for support.
However, data quality may be affected by various factors and problems may arise.
In this survey, 80% of financial institutions reported that after the model was launched, the computational efficiency and stability of the model were affected by changes in processed data.
Second, in the face of diverse fraud tactics, models cannot be universally applied.
Anti-fraud models based on machine learning require a significant amount of time and data samples to train, optimize, and maintain for a single type of fraud.
Once the fraud tactics change, the model cannot adapt automatically and needs to be retrained, developed, and launched again, during which a large number of fraud incidents may occur.
For example, the recent shift towards "self-operation" in fraud trends has made the boundary between normal and abnormal transaction data increasingly blurred, leading to a gradual decline in model performance and occurrences of false positives and false negatives.
The survey found that more than 80% of institutions reported that model maintenance and updates are the main difficulties currently existing.
Finally, to improve the efficiency and security of verification, financial institutions' anti-fraud verification rules are gradually shifting from traditional manual verification to digital and intelligent verification.
However, from the current situation, the results of system and big data collaborative verification often do not match manual verification and cannot be directly applied to actual work.
In addition, some types of verification involve a large amount of invisible information that cannot be directly obtained through the system and can only be verified manually.
4.
The high cost of system platform construction is difficult for small and medium-sized institutions to bear.
In recent years, there has been a trend for fraud gangs to shift their account opening targets from large banks to small and medium-sized banks and third-party payment institutions.
The dark and grey industry takes advantage of the relatively weak risk control of small and medium-sized institutions to reduce its own exposure risk.
However, for small and medium-sized financial institutions, due to relatively fewer funds and account scales involved in cases, insufficient accumulated data, and a lack of composite talents, it is difficult to invest sufficient resources in the construction of anti-fraud system platforms.
Faced with a severe risk situation, in addition to continuously strengthening their own technological capabilities and coordinating the construction of anti-fraud systems, small and medium-sized financial institutions also urgently need to access plug-and-play and highly agile inclusive anti-fraud data service products.
1.
Improve the top-level design of sub-domains and accelerate industry data sharing.
First, data sharing standards and specifications should be perfected as soon as possible.
Relevant departments should take the lead in top-level design, establish a sound data sharing standard system, and introduce more comprehensive privacy and data security policies to eliminate institutional concerns.
At the same time, it is also necessary to promote the formulation of baseline standards such as privacy computing-related security testing standards, performance testing standards, cryptography evaluation standards, and interconnectivity technology standards.
Based on existing security grading standards, explore the formulation of security levels for individual privacy computing technology routes, as well as general security grading standards and security assessment standards for privacy computing that integrates across technologies.
Second, a data sharing practice guide should be issued.
Establish data indicators and technical interface standards as soon as possible to guide the resolution of difficulties in information sharing and connectivity between industries.
Establish a data sharing evaluation index mechanism to fully mobilize the subjective initiative of institutions, thereby achieving collaborative linkage in anti-fraud work.
Accelerate the introduction of financial application guidelines for privacy computing, supplement the description of relevant legal and regulatory provisions, provide policy guidance for the compliance application of privacy computing in the field of anti-fraud, and clarify the provisions on data security grading and regulatory audit for privacy computing, to enhance the level of industry technology application.
2.
Lead management in multi-party cooperation to promote the construction of anti-fraud platforms.
There is a necessity to build an industry anti-fraud platform.
First, anti-fraud, due to its social welfare attributes, needs to be jointly completed by management departments, public security, operators, and other participants in the joint prevention and treatment of anti-fraud.
Second, small and medium-sized financial institutions, due to the incompleteness of data accumulation and resources, find it difficult to invest sufficient resources in anti-fraud construction.
Third, the dark and grey industry is good at discovering and targeting the weak points of risk control construction in different regions and institutions for concentrated attacks, and once successful, the speed of fund transfer is very fast.
Under such circumstances, the individual efforts of financial institutions cannot fully suppress fraudulent behavior.
Therefore, the industry should explore the joint construction of an anti-fraud platform, to popularize and equalize anti-fraud services in the form of public products and services.
In terms of functionality, the survey results show that blacklist sharing and fraud intelligence sharing are important functions that financial institutions hope the platform can have, followed by real-time or quasi-real-time query functions and guidelines and suggestions for the application of rules and models.
In terms of application mechanisms, financial institutions are most concerned about the security and confidentiality of their own commercial secrets and customer privacy, followed by data sharing incentive mechanisms, and whether the platform application can meet regulatory and compliance requirements.
3.
Formulate risk control management strategies and establish a good risk culture.
Financial institutions should first formulate management strategies from a strategic height, strictly implement various regulatory requirements, improve the risk management system for fraud, and promote the improvement of a full-process risk management system that covers the identification, assessment, detection, control, and reporting of fraud risks.
At the same time, it is necessary to strengthen the construction of anti-fraud and risk control teams, establish an anti-fraud prevention and control structure based on risk, business, and technology, and continuously enhance the professionalism and agility of the business security team in dealing with fraud risks.
Only by establishing a good internal risk culture and strengthening the risk awareness of all employees can the institution better cope with the challenges faced by business security and ensure that risk control work is carried out smoothly and effectively.
4.
Keep up with the wave of AI development and enhance intelligent risk control capabilities.
First, AI can achieve more accurate user tagging and preference recognition, helping financial institutions establish more precise user portraits, thereby assisting in user risk identification.
Second, in terms of deep data mining and user relationship analysis, AI technology can deeply mine the bank's own asset data, provide a visual relationship network, and empower business personnel to conduct user association analysis and gang risk analysis more intuitively and quickly.
Third, AIGC can be used in the field of bank fraud identification, such as fraud monitoring, intelligent customer service, identity verification, anti-money laundering, and anti-terrorism financing, and other scenarios.
Due to the current existence of low explainability, high costs, data privacy, and security issues, the application of large model technology in the field of financial anti-fraud is still a certain distance away, but the application prospect of this technology is good, the model has strong identification capabilities, and it can be continuously explored.